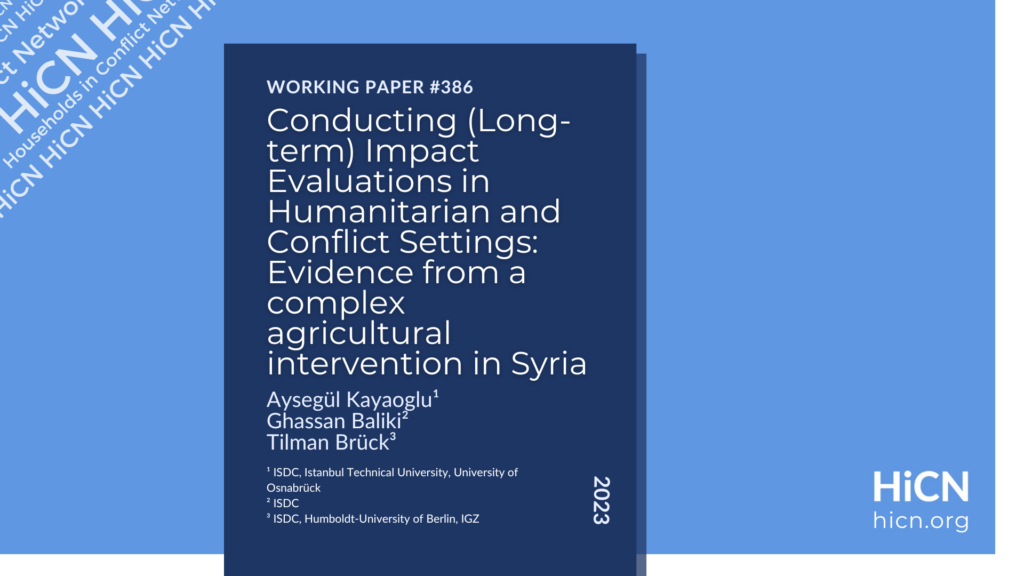
The number of vulnerable people in humanitarian emergencies worldwide is increasing due to the rising frequency and intensity of risk exposure. At the same time, most interventions in humanitarian emergency and conflict settings (HECS) are short-term in nature, as if people only require temporary help to overcome adversity. Yet there is an acute scarcity of rigorous impact evaluations in HECS testing if assistance works well (or at all). Moreover, the few available studies only cover a small range of countries and contexts. Furthermore, the knowledge gap concerning the long-term impacts of crisis interventions is even more pronounced. These gaps are primarily caused by the unavailability of (long-term) panel data in emergencies and by the challenges of constructing feasible counterfactuals. Our paper contributes to the literature in four ways. First, we review recent research on covariate balancing to assist researchers in conducting a rigorous impact evaluation in HECS with non-randomized treatment assignments and significant covariate imbalances between the treatment and control groups due to targeting. We thus suggest methods to overcome the challenges associated with conflict or humanitarian contexts. Second, employing a range of such methods for one case study, we offer rigorous evidence on the long-term causal impacts of agricultural interventions in a humanitarian crisis setting. Third, we show that agricultural or livestock interventions have different impacts in the long term, which implies that the combined interventions might have a more sustainable impact on households. In other words, our analyses demonstrate that short-term humanitarian assistance can indeed have long-term development impacts. Fourth, we offer innovative evidence for the case of Syria, using unique panel data with four waves of treated and untreated households, thus expanding the range of countries ever studied in the literature on humanitarian emergencies.